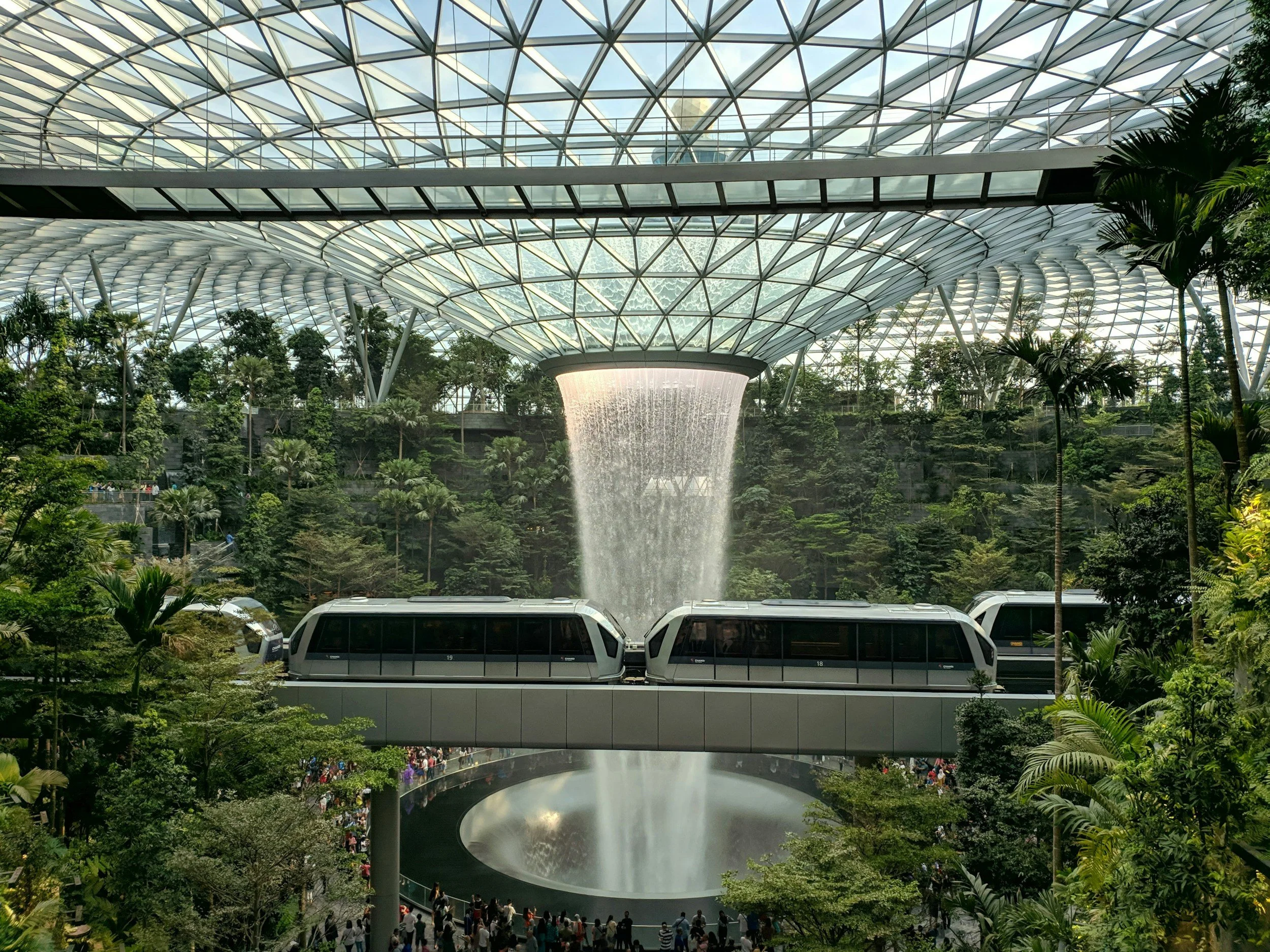
Blog
Ideas That Spread
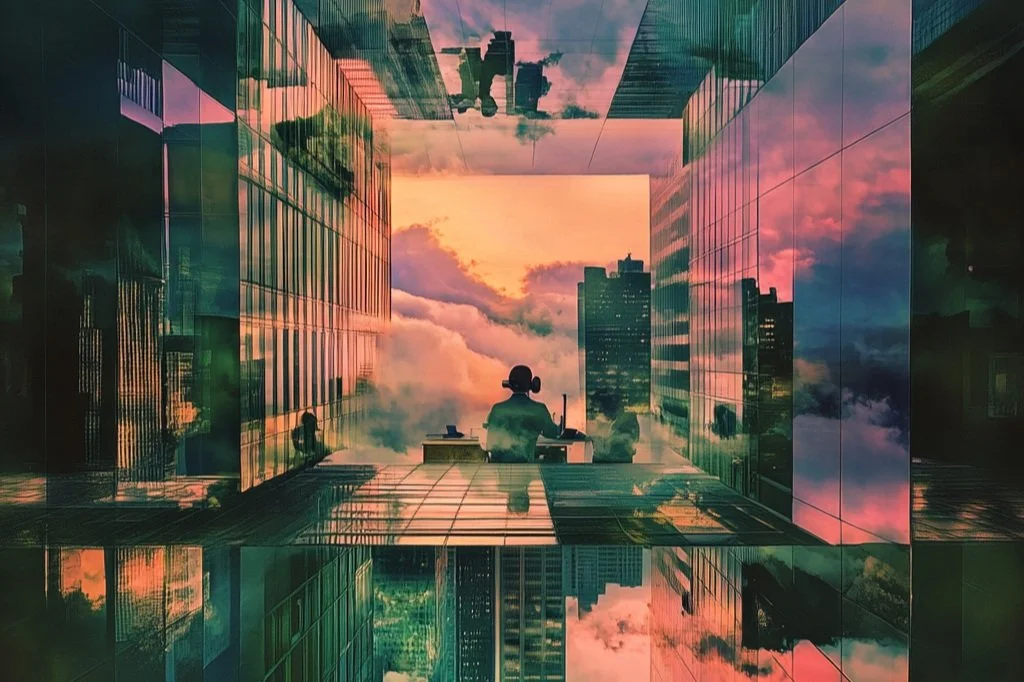
What Does a Fully AI-Integrated Business Look Like?
Despite AI's potential to transform industries, many companies still struggle to implement it successfully across their entire business. Gartner reports that only 15% of enterprises have integrated AI into multiple processes, with many companies still stuck in the pilot phase. Challenges range from outdated infrastructure to a fundamental lack of AI expertise. But the real barrier often boils down to one thing: trust.
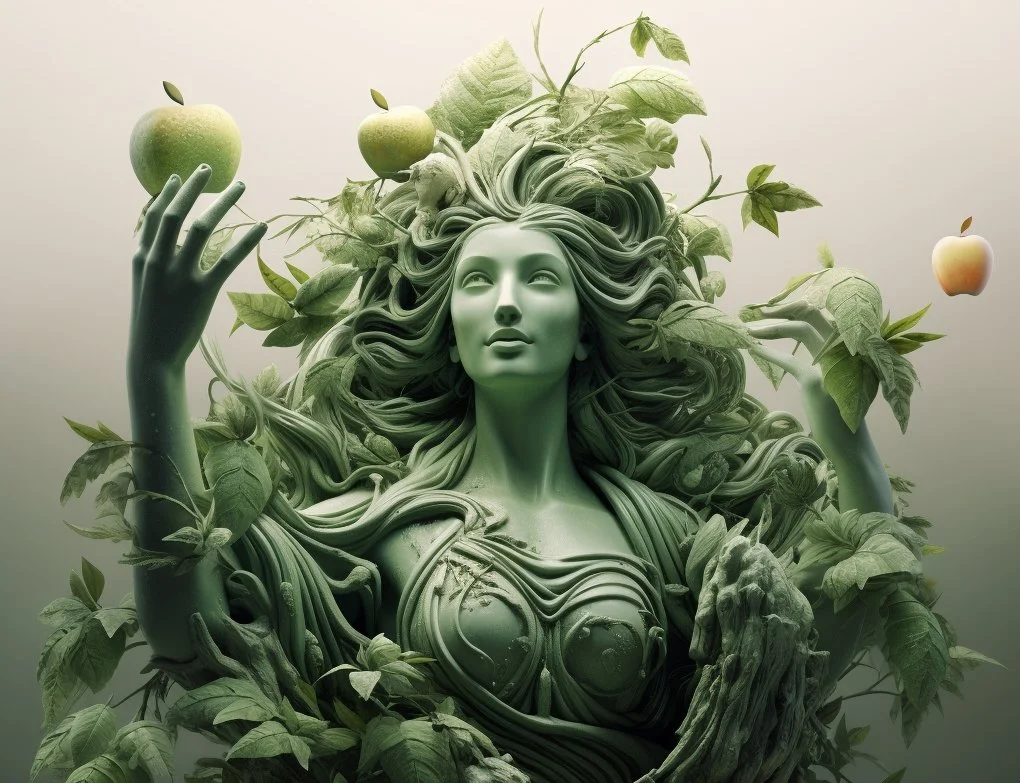
What is a “Living Brand”?
In the ever-evolving world of branding, the concept of a "living brand" emerges as a beacon of innovation and responsiveness. A living brand is akin to a sentient being; it possesses the ability to sense, empathize, decide, act, and evolve. It is adept at gathering and processing data to understand and respond to customer needs, recognize emotions, make valuable decisions, and learn from past outcomes to enhance future outcomes.
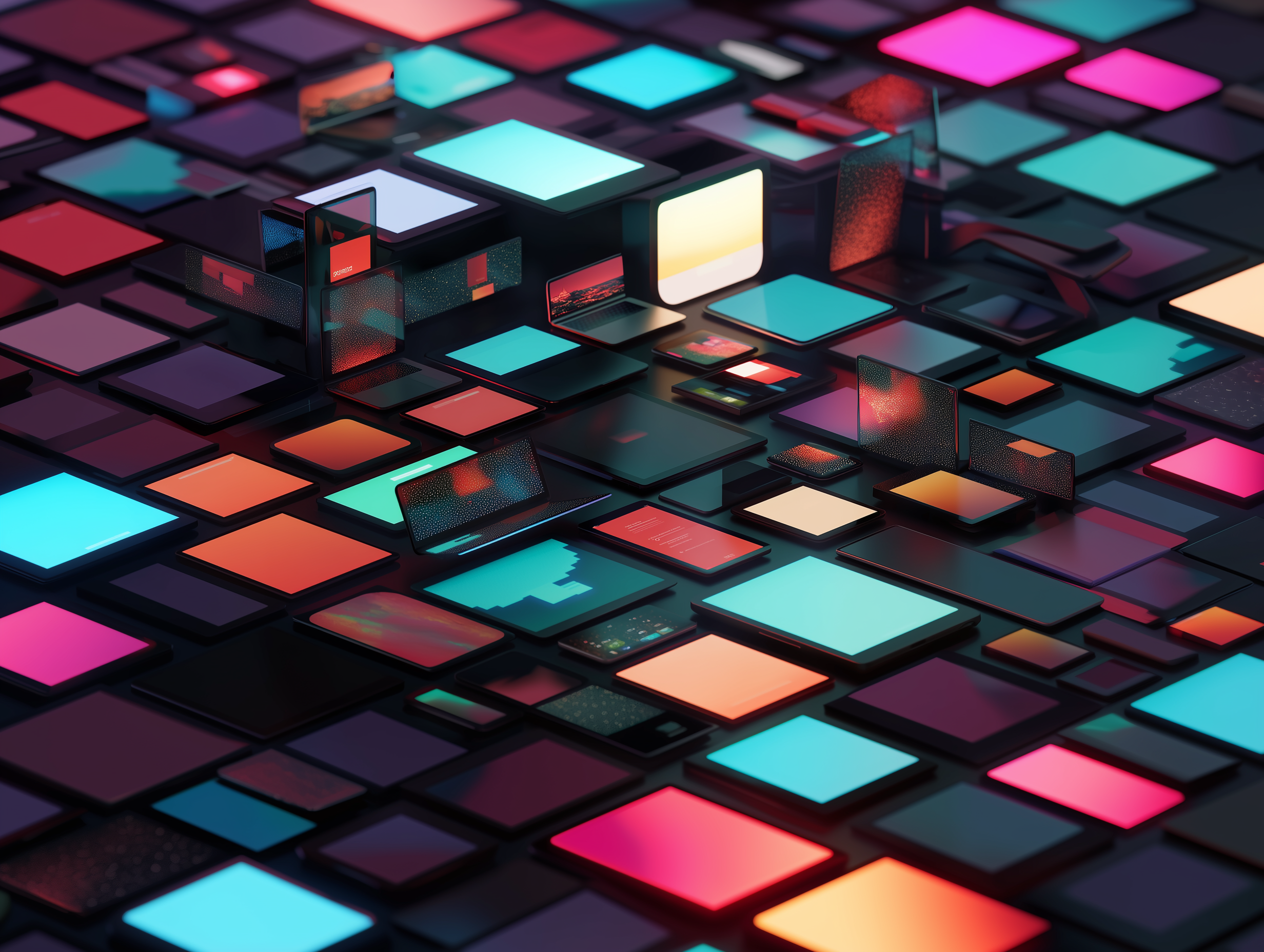
How Brands, Agencies, and Creatives Are Using AI to Elevate Content Strategies
Artificial intelligence (AI) is reshaping content creation, offering new ways for brands, agencies, and creatives to push the boundaries of storytelling and engagement. However, AI is not just about automation—it's a tool that can support human creativity, helping teams work more efficiently while maintaining the core identity and strategy of each brand. Here’s how AI is empowering content creators and marketers to produce more impactful work:
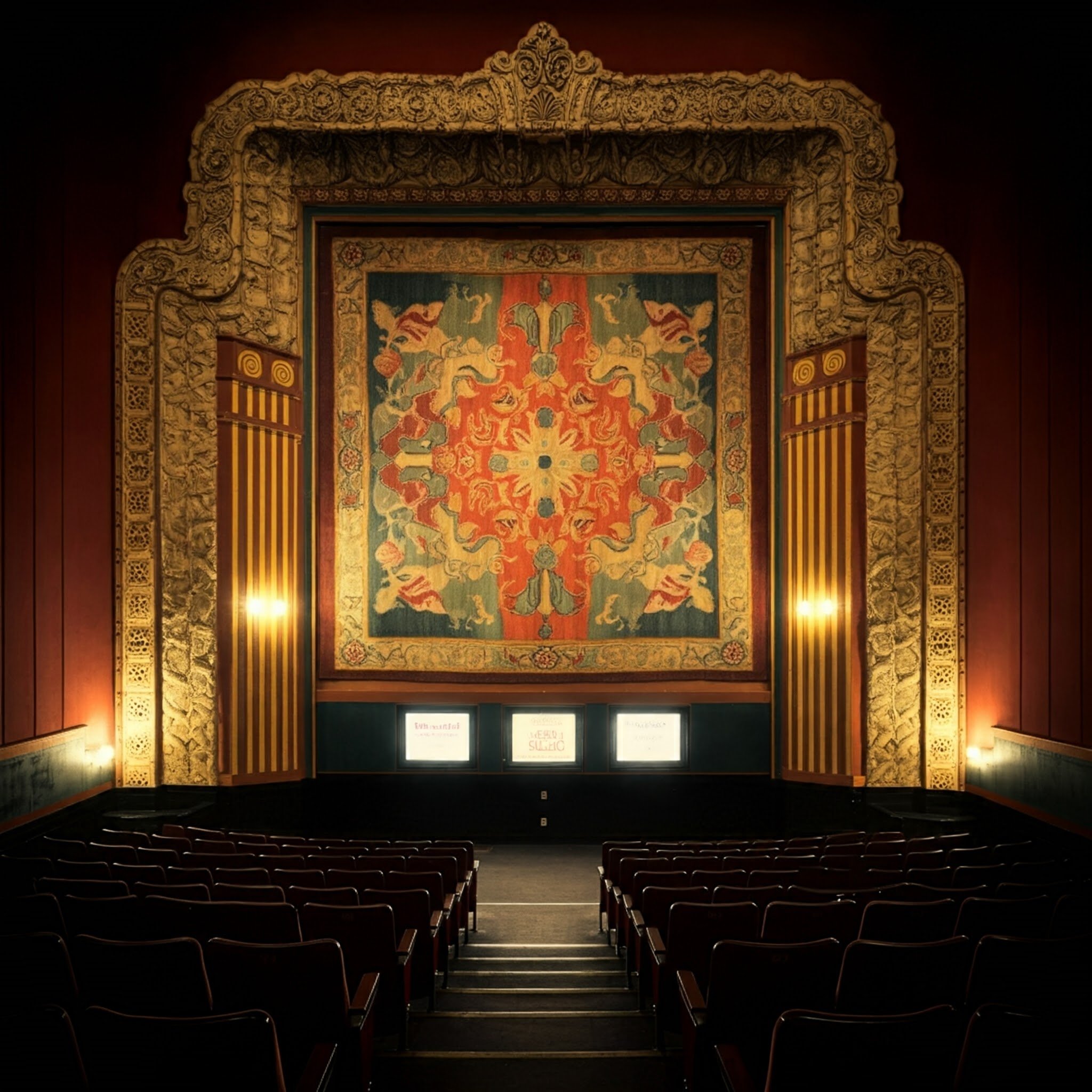
And the Award for AI Word of the Year Goes To… Tapestry
Lately, I’ve noticed something odd in branding. Every company is weaving its story into a “tapestry.” Yes, tapestry. It’s as if AI decided this was the magic word to convey elegance, complexity, and sophistication. Suddenly, every mission statement is a “tapestry of values” or a “tapestry of innovation.” If AI had a Word of the Year, tapestry would win by a landslide.
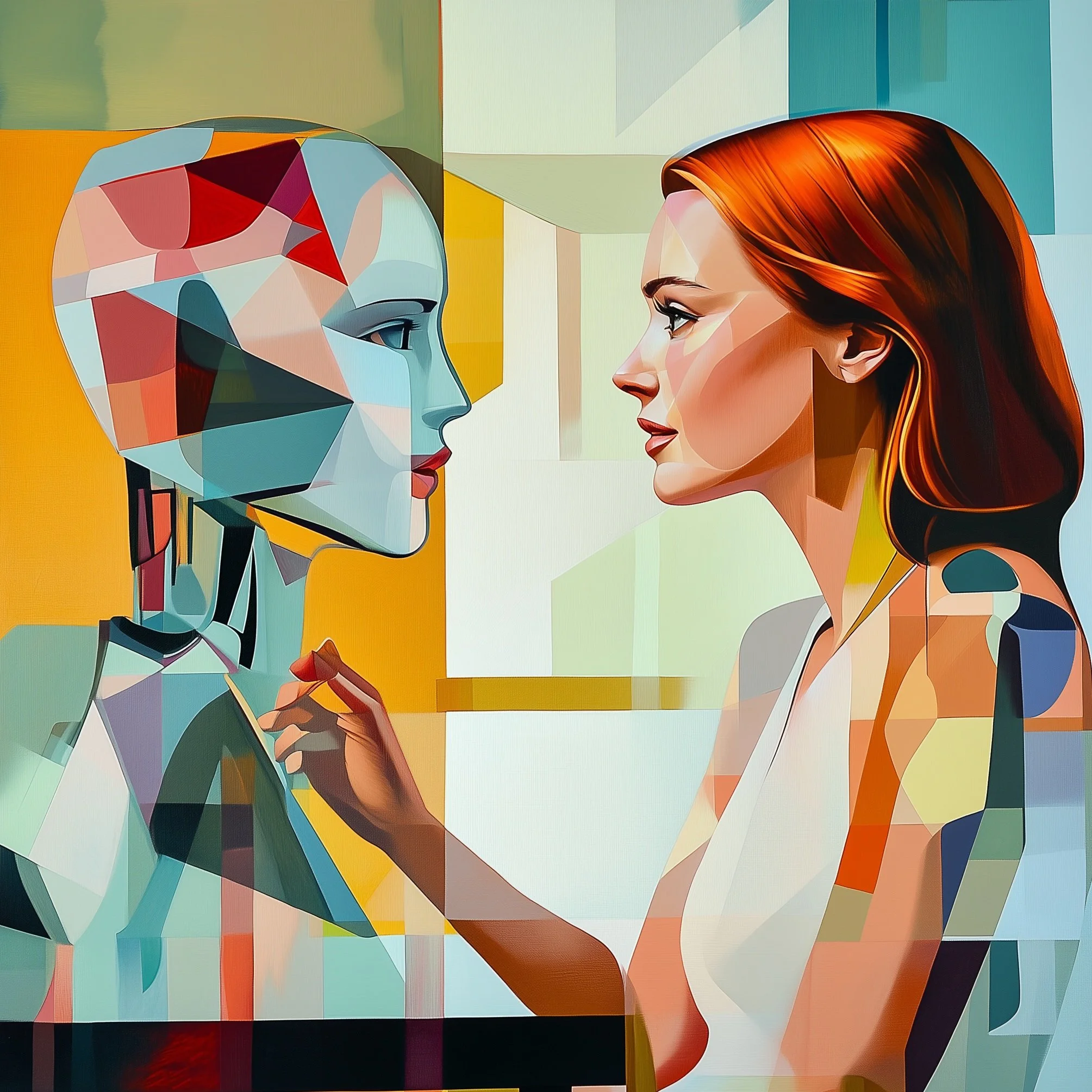
"The Résumé Revolution: Standing Out When Everyone Sounds the Same"
Lately, I've noticed an eerie similarity between my résumé and those of others in my network. Case in point: a colleague recently shared her professional bio on LinkedIn, and as I read it, I had a-ha moment. It was as if she had copy-pasted my résumé! (Of course, she didn’t, but the uncanny resemblance was there.) And it's not because we lack originality; it's because AI résumé tools are crafting everyone's stories from the same algorithmic recipe book.
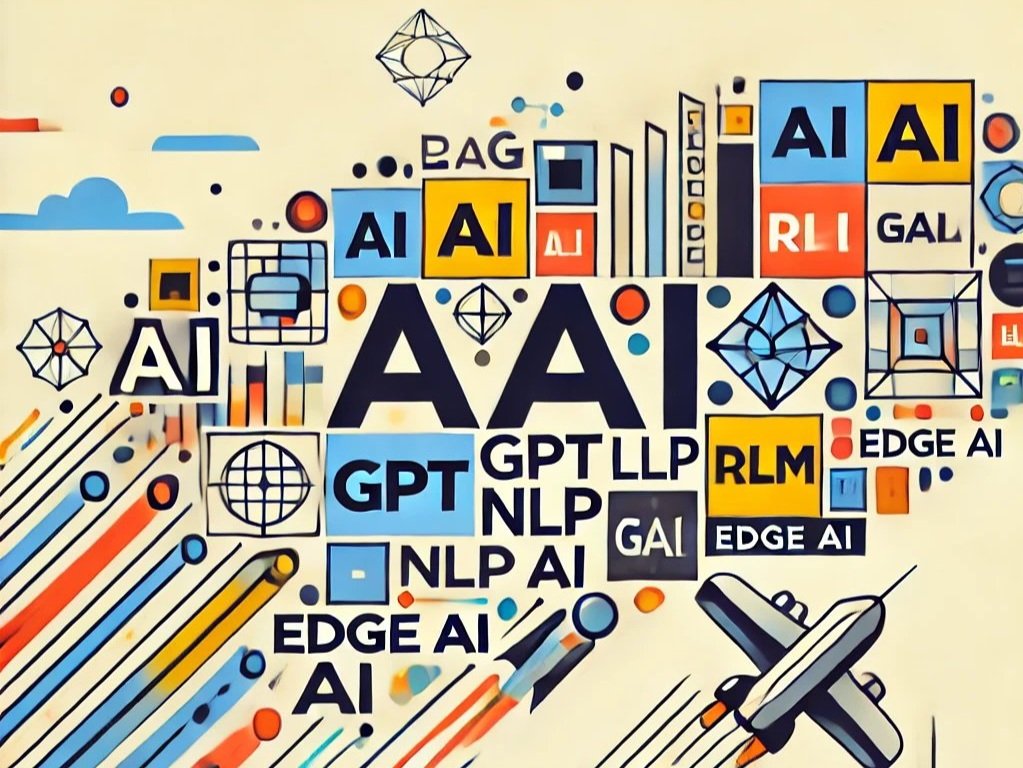
AI Stands for Artificial Intelligence, But What’s a GPT or an LLM??
Let’s be honest—how many AI buzzwords and acronyms do you really get beyond AI meaning Artificial Intelligence? Maybe you’ve heard of LLMs (Large Language Models) or know that a Neural Network has something to do with how machines "learn," but for most people, these terms fly right over our heads. Just like when www became part of our vocabulary or when the language of the .com internet exploded into the world, we had to learn a whole new language: megabytes, pixels, bandwidth. Sound familiar?
